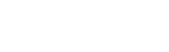
An Adkodas Experience
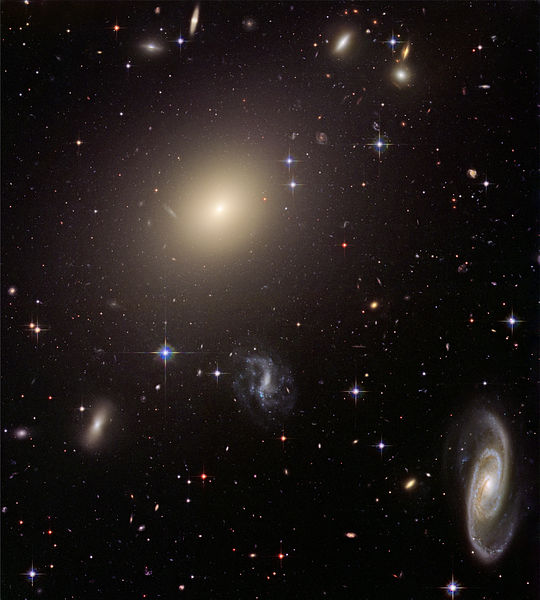
During my PhD at Stanford, part of my thesis involved the mass-classification of galaxies from large digital surveys: at its most basic level, was a given galaxy a spiral galaxy or an elliptical galaxy? Such a classification of morphological type (much less a more detailed one) is necessary in order to investigate the evolution of galaxies over time, both within and between types. Yet, with billions of objects pushing the boundaries of what is meant by "Big Data," such surveys have been for some time well beyond the old-fashioned process of graduate students visually classifying images "by eye" for an entire data set.
The challenge? To find an automated way of classifying galaxy type. There are, of course, models for classifying galaxy types--but such models often rely on many data points per object being available, which may not be true across an entire catalog, and especially for the more distant objects it contains. While existing neural networks can be trained to produce results, such networks operate under a train-and-check method that allows a computer to make a statistical guess about each object, but offers little enlightenment as to what data is most useful in classifying it.
Large surveys contain many fields of data that may or may not correlate with galaxy type, and while my advisor's model had some predictive value--dependent on the availability of several reported light-profile measurements per galaxy--for many galaxies, especially the most distant, it was unable to clearly separate spiral types from ellipticals, much less any finer demarcations.
Using early Adkodas technology, I was able to find in the data an unexpected field--one that would not seem to have any particular correlation to galaxy type--and not only that it was a better predictive value than existing models, but I was able to go back and calculate what the value was and *why* it was a better predicter than the standard models.
(In short, the unexpected field was an automated-object-size-detection field, a Gaussian full-width-half-maximum. In the catalog, this was only an intermediate step for calculating detected object size, but with the Adkodas results in hand, I was able to calculate why the Gaussian profile fit the expected shape of a spiral galaxy differently than it fit the expected shape of an elliptical.)
Technicalities aside, existing classification methods, such as that presented to me by my advisor, with intensive effort and needing multiple data points per object, only correctly classified 50-75% of galaxies, depending on type; Adkodas-indicated and independently-verified data fields, when combined into a new, simple two-parameter model, immediately and correctly classified 85% of each galaxy type.
This discovery proved to be the most interesting, most "mine" part of my thesis, a genuinely new and exciting discovery and one that I could back up with my own calculations and not just "the computer says so."
At the time, the Adkodas process of directly finding rules within data sets was still manual and painstaking, if rewarding. Since then, Adkodas technology has advanced to improve the automation of finding rules, so that you, too, don't have to rely on a black box to give you an answer--instead, you can investigate why.